ISL_Chapter6_Exercises
Written on April 26th, 2018 by hyeju.kim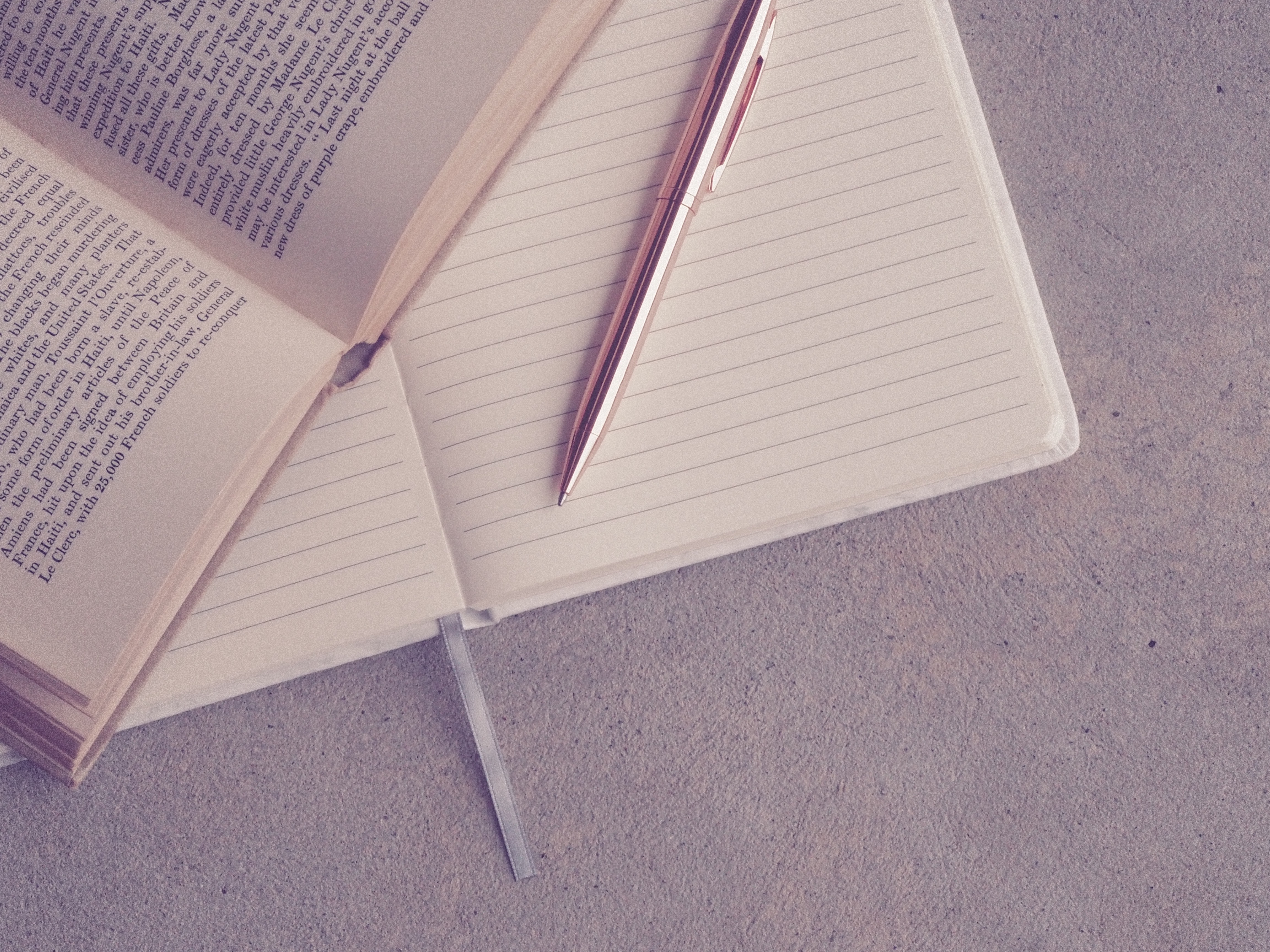
Chapter6 Exercises
Conceptual
-
(a) best subset method. It decides best way through making all the possible models.
(b) best subset method. The reason is the same with above.
(c) i. True. subset + (k+1) variable or not
ii. True.
iii./iv. False. backward and forward could have different subset
v. False. because the best way could be changed with adding the (k+1) variable or not.
http://people.stat.sfu.ca/~lockhart/richard/350/08_2/lectures/VariableSelection/web.pdf
-
(a) Lasso iii-True. Lasso regression decrease variance by limiting the variance of estimated coefficients(less flexible),but the bias could increase
(b) Ridge iii-True. same with Lasso.
(c) Non linear ii-True. flexible method increase variance, but decrease bias.
-
if s becomes larger, penalty according to the variance of coefficients is smaller. increase variance and decrease bias.
(a) training RSS iv because when s=0, all coeffiecients are 0, then RSS would be maximum and, then when s becomes larger, RSS would be decreased.
(b) test RSS ii correct (variance increases and bias decreases as s becomes larger) (over simple to over fitting)
(c) variance iii correct
(d) bias iv correct
(e) irreducible error v correct: By definition, irreducible error is model independent and hence irrespective of the choice of ss, remains constant
-
if $\lambda$ becomes larger, penalty according to the variance of coefficients is larger. decrease variance and increase bias.
(a) training RSS iii correct because when $\lambda$ becomes larger, RSS would increase because its coefficients are limited by penalty.
(b) test RSS ii correct ( bias increases and variance decreases as s becomes larger) (overfitting to over simple)
(c) variance iv correct
(d) bias iii correct
(e) irreducible error v correct nb\
]
-
(a)
(6.12) becomes $(y_1- \beta_1)^2 + \lambda\beta_1^2$ with p=1
$(y_1- \beta_1)^2 + \lambda\beta_1^2$
$= (\lambda+1)\beta_1^2 - 2y_1\beta_1 + y_1^2$
is minimized when $\hat\beta_1 = y_1/(1+\lambda)$ when $\lambda>0$
(b)
(6.13) becomes $(y_1- \beta_1)^2 + \lambda | \beta_1 | $ with p=1 |
if $\beta_1 > 0$
$(y_1- \beta_1)^2 + \lambda\beta_1$
$= \beta_1^2 - (2y_1-\lambda)\beta_1 + y_1^2$
is minimized when $\hat\beta_1 = y_1-\lambda/2$ when $\lambda>0$
if $\beta_1 < 0$
$(y_1- \beta_1)^2 - \lambda\beta_1$
$= \beta_1^2 - (2y_1+\lambda)\beta_1 + y_1^2$
is minimized when $\hat\beta_1 = y_1+\lambda/2$ when
if $\beta_1 = 0$
모르겠어여…